Five Ways AI And Machine Learning Can Enhance Cybersecurity Strategy
Artificial Intelligence (AI) and its essential component machine learning are causing a stir in practically every industry from marketing to education. It’s no wonder designers and tech developers are finding ways to use the benefits of automated technologies to improve cybersecurity infrastructure and defend against increasingly complex and numerous cyber threats. That being said, AI is not a cure-all for all cybersecurity threats. At the end of the day, it’s a piece of technology, a tool designed to help companies work better, so, like any tool, it has to be applied with consideration if it’s going to work. With that in mind, here are some ways in which AI (or, more precisely, machine learning) is being used to enhance cybersecurity measures.
Robot Wars
“One of the reasons machine learning is so useful in combatting cyber attacks is that the attackers are already using machine learning against you,” says Flora Oglivy, a cybersecurity specialist at Writemyx.com and Nextcoursework.com. “Cybercrime using AI technology is so powerful because it’s tireless, it can continually test defenses until they find a chink in the armor. Employ machine learning in your defenses, however, and suddenly you have an indefatigable security guard to defend against the indefatigable thief.”
Marking Out Malware
Another use case for machine learning is in altering you to the presence of malware. Thanks to the work of thousands upon thousands of cybersecurity professionals over lifetimes of work, we have a good amount of examples of malware at our fingertips. Once a new example is discovered it’s easily labeled as malicious technology and can be removed from a system. Machine learning thrives on this kind of data: a simple yes or no question based on finding characteristics. As a result, supervised machine learning systems are great for sorting between regular applications and malware versions and can alert administrators whenever a malicious program is identified.
Sniffing Out Spam
The same concept goes for spam identification technology. Mark Chapel, a tech blogger at Britstudent.com and Australia2write.com, asks “have you ever wondered why your mail carrier can identify spam emails? The answer is: machine learning. Algorithms trained on typical spam emails help to identify unwanted messages and automatically sort them out of your inbox. That means, whenever you mark an email in your inbox as spam, you’re actually helping to train the bot.” Google’s spam filter is a good example of a powerful machine learning technology in action. According to a study last year, the search engine employs hundreds of rules to sort spam from regular emails, using optical character recognition (OCR) to identify keywords and phrase structures common across spam emails.
Scalable Technology
With machine learning technology the sky’s the limit, meaning they’re able to scale with a company’s security needs as the data they handle grows. In fact, the longer an AI system is in place and the more data it handles, the better at its job it will get, meaning investing in a machine learning aspect to your cybersecurity strategy is only going to pay back dividends as time goes on. I say the sky’s the limit, but that’s only hypothetically; in practice, the limit is data storage. Thankfully, machine technology lives very comfortably in the cloud, so storage space becomes less of a problem and the system can continue to expand unimpeded.
Tools, Not Cures
All of this notwithstanding, If you hope to install a machine learning system to learn your attackers’ moves and combat their breaches by itself then you’ve not understood the point of AI. All of these applications are not magic fixes, they require significant work to set up and maintain, which is why any AI solution is a tool, not a cure. Perhaps at some point in the future, we can develop AI machines powerful enough to create other AI machines, at which point we may well be looking at the singularity end of existence. However, until then, machine learning relies on clever applications and dedicated programmers. The technology is practically a blank slate, how you apply it depends on your cybersecurity needs and the data you train the system on.
Katrina Hatchett is a tech blogger at Academic Brits and writer for Essay Writing Service. She works with companies to identify and solve project issues, and her goal is to improve the effectiveness of our communication. She also writes for PhDKingdom.com about the future of machine learning.
Most Recent Articles
- A beginner-friendly intro to the Correlator for effective cybersecurity detection
- Inotify in ASAB Library
- From State Machine to Stateless Microservice
- Entangled ways of product development in the area of cybersecurity #3 - LogMan.io
- Entangled ways of product development in the area of cybersecurity #2 - BitSwan
You Might Be Interested in Reading These Articles
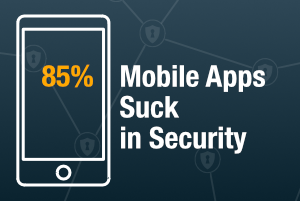
We Know Why 85% of Mobile Apps Suck in Security. Do You?
In just the past 12 months, we’ve come across 100 mobile app projects at different phases. We’ve had conversations with more than 300 professionals active in the enterprise mobility space. We asked questions and uncovered the underlying problem that caused the current miserable state of mobile application security. It sucks. The answer doesn’t lie in technology but in us.
Published on May 19, 2016
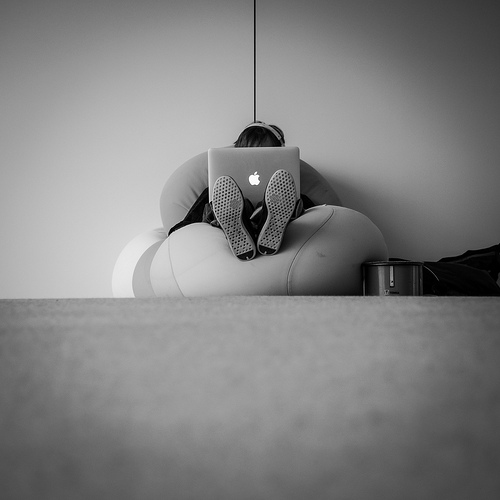
SQL Injection - Are Developers to Blame for Data Security Breaches?
Of course, this is a bold statement, but for those who deal with security issues from mobile applications, they can pinpoint where the flaw occurred with developers not taking security into account when developing mobile apps. Security takes the back seat to app functionality and remains as second thought.
Published on March 07, 2015
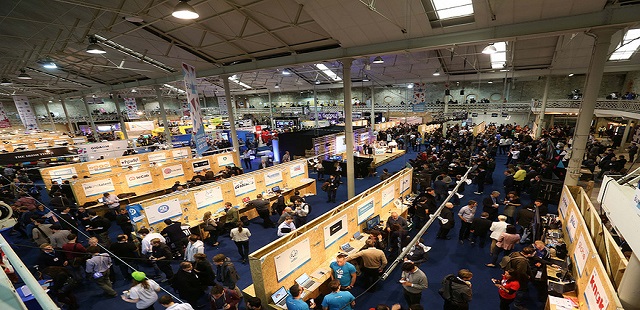
How to Win in the Enterprise Mobility Market
At the Dublin Web Summit, I had many interesting chats with people who developed mobile apps for enterprises and large companies. Despite their differences, most had the same mindset regarding the security of their mobile apps and the backends. 'It's as secure as the infrastructure at our customers.' Is it? Let's find out.
Published on April 21, 2015